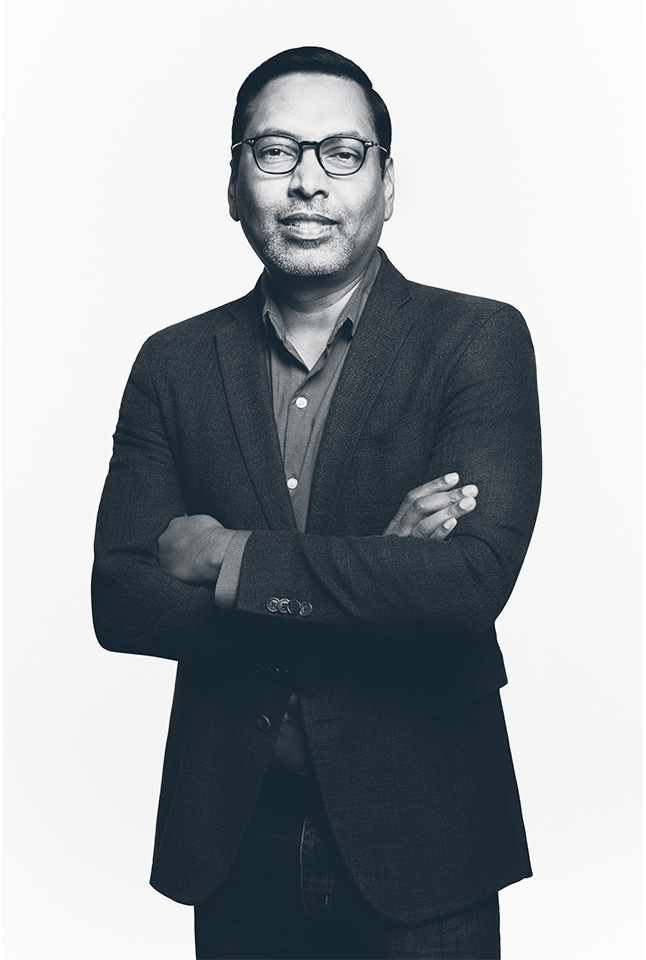
Investor POV: AI governance and responsible use
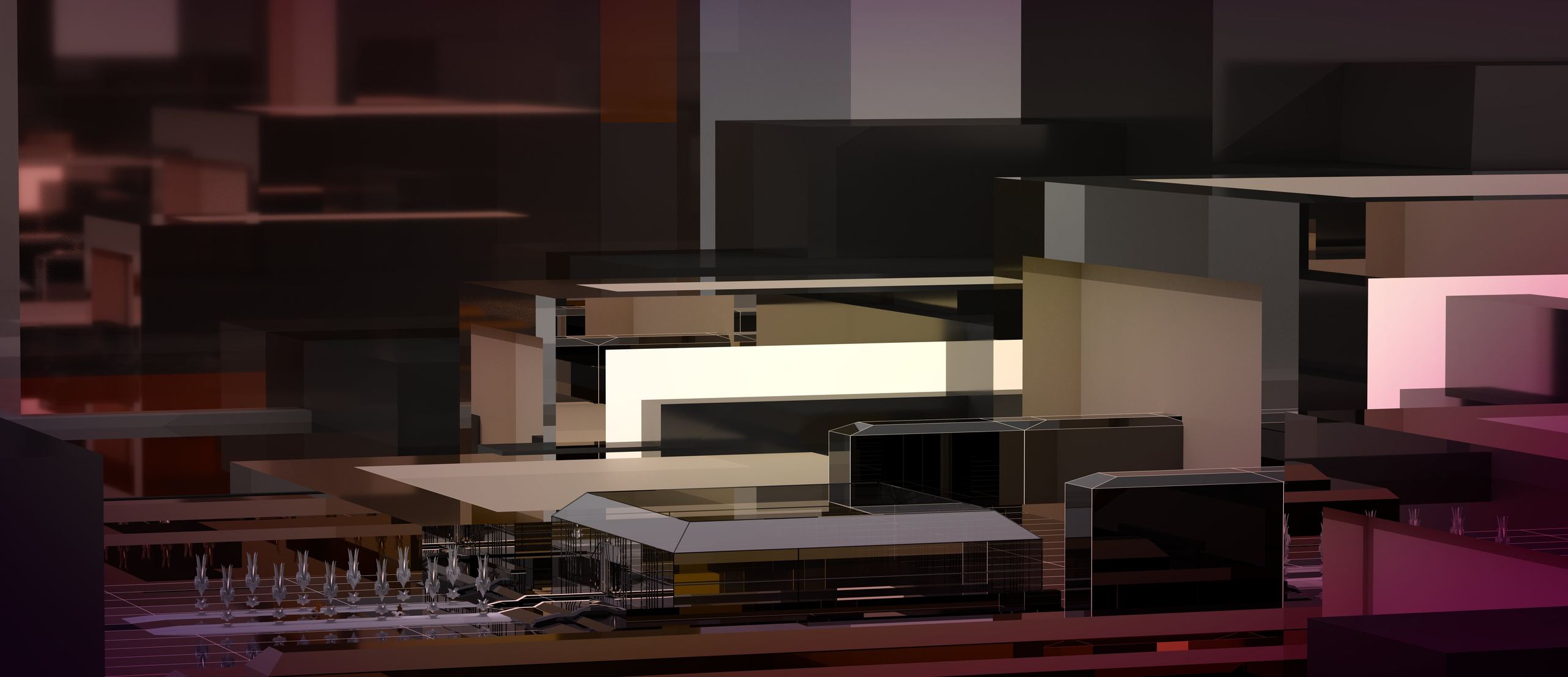
In 2023 we’ve seen a frenzy of innovation in AI – from the proliferation of large language models (LLMs), to the scale of adoption of ChatGPT, to the rapid evolution of the MLOps infrastructure layer. These transformational innovations seem poised to disrupt nearly every business in nearly every industry, and both startups and enterprises are scrambling to integrate AI into their product strategies and go-to-market (GTM) playbooks.
But as companies race to deploy AI, discussions of risk are starting to take center stage. Regulatory pressures are mounting, business and technology leaders continue to issue warnings about potentially existential AI risks, and a full ⅔ of enterprise executives admit that they have reservations about being able to use AI ethically, responsibly, and compliantly. The moment has come for business leaders to ask not just how do we adopt AI, but how do we adopt AI in a way that is safe, reliable, and aligned with human values?
The moment has come for business leaders to ask not just how do we adopt AI, but how do we adopt AI in a way that is safe, reliable, and aligned with human values?
This post will explore recent developments in AI Alignment, Management, and Safety (a key segment of layer 3: ‘Build’ in the below graphic), focusing on the quickly evolving regulatory landscape and highlighting companies that are helping enterprises ensure their AI strategies are responsible. As our survey of the AI governance ecosystem will reveal, despite the urgent need for AI governance solutions, the landscape of software tools helping enterprises deploy AI safely is nascent.
We believe there is a huge opportunity to help enterprises operationalize AI regulation and build robust AI governance strategies.
The rapidly evolving AI regulatory landscape
AI regulation is developing rapidly across the world, with many governments and NGOs rushing to pass laws and build frameworks that will help mitigate risk and ensure AI is responsibly deployed.
At the center of much of the global dialogue on AI regulation is the EU AI Act, which is on track to be finalized no later than December 2023. The law will require ‘high-risk’ companies with AI deployed in fields like education, employment, and law enforcement to provide detailed information about their AI systems, focusing on foreseeing potential risks to health, safety, fundamental human rights, and discrimination. Substantial fines are starting to get enterprises’ attention – penalties for violators are set at 4% of annual global revenue or €20m, whichever is higher.
Outside of the EU, regulators are working hard to design and publish guardrails – from the US National Institute for Standards in Technology’s voluntary AI Risk Management Framework to Singapore’s Model AI Governance Framework to the UK’s White Paper on AI.
While governance approaches differ across nations and will continue to evolve along with frontier innovations in machine learning, one thing is clear: the era of unregulated enterprise AI is coming to a close. Businesses will soon be compelled to produce detailed information about the monitoring and control of their AI systems, or they will face significant consequences.
Key example: Algorithmic bias in hiring
It’s in this context that we want to examine the current state of play for where alignment, management, and safety of AI models stands today. Much of the emerging AI regulation focuses on auditing algorithms for bias. This focus is natural, as many of the early AI models deployed by enterprises have had public issues with discrimination.
Take Amazon as an example: between 2014 and 2017, the tech giant tried to build a tool to review resumes and streamline their recruiting process. After training the model on ten years of historical hiring data, the algorithm ranked resumes that included the word “women” (e.g., a women’s sports team) as weaker than resumes that included “men.” The project was dropped after attempts to fix the model’s bias failed. But it’s not just Amazon – many resume screening tools face similar problems. Perhaps most pointedly, one candidate review platform suggested that having the name ‘Jared’ was among the strongest indicators of high job performance.
Off the back of these incidents, legislation is beginning to emerge to prevent biased models from reproducing and exacerbating social inequalities. NYC’s LL-144, for example, prevents employers from using automated employment decision tools (AEDTs) in hiring and promotion decisions unless the platforms have had an annual independent bias audit that assesses disparate impact based on race, ethnicity, and sex. The law went into effect this month, and while there is ongoing debate over exactly which tools fall under LL-144’s scope, New York companies are beginning to prepare for audits.
Investor POV: What We’re Excited About
As regulation like LL-144 pushes ahead across continents, startups are emerging to help enterprises keep track of rapidly changing policy developments and ensure that their AI strategies comply with regulation. But despite the urgent global need for responsible AI tools, the landscape of AI governance solutions is nascent.
We believe there is a significant opportunity for startups to help enterprises build their AI governance programs – and that both purpose-built AI governance tools and MLOps tools will be critical parts of enterprises’ responsible AI strategies.
Purpose-built AI governance platforms
Several governance platforms have emerged in the last few years that are focused on helping enterprises deploy AI responsibly. For example, Credo, Monitaur, Holistic, Trustible, Vera, and Conductor all offer horizontal AI governance products that help enterprises identify the potential risks in their current AI programs and operationalize forthcoming AI policies. Most of these tools serve business stakeholders (as opposed to technical stakeholders) and focus on giving businesses a high-level overview of their AI systems. They answer questions like ‘Where is AI deployed across my organization?’ and ‘Do my AI systems meet technical requirements set by regulators around bias and fairness?’. As AI continues to proliferate across enterprises, we believe these tools will serve as an essential first line of defense in AI governance, helping enterprises map potential risks across the organization and understanding where more attention needs to be directed.
But identifying potential AI risk is just the start. As regulation matures and enterprises see that many of their AI programs do not meet regulatory requirements, attention will shift from merely understanding if models comply with regulation to understanding how to build models that comply with regulation. In this vein, we are excited about vertical-specific solutions that are focused on building compliant ML models, particularly in high-risk industries like financial services and insurance. Zest, for example, offers an AI-driven credit underwriting product that helps lenders explain, document, and validate their lending decisions. Before Zest, many lenders struggled to leverage cutting-edge machine learning techniques – the models were considered too “black box” to meet regulatory standards around explainability (and would fail the technical assessments that platforms like Credo and Vera help enterprises run). Zest’s explainable credit model now allows banks to deploy sophisticated ML models that drive meaningful financial ROI and pass regulatory standards. We believe there is a significant opportunity for durable company creation in other highly regulated industries like health care and law enforcement, where vertical-specific, explainable AI systems can be built on the back of large, sensitive data sets.
Model monitoring and feedback
In addition to purpose-built responsible AI platforms, we are excited about the role that MLOps tools will play in the emerging AI governance stack, particularly those focused on model monitoring and feedback. Because many of the critical challenges in AI governance bridge social and technical questions, we believe that comprehensive AI governance strategies will depend on integrated, cross-functional solutions. While AI governance platforms will be critical in helping enterprises articulate what kind of values they want to instill in AI systems, MLOps platforms will be necessary to translate and align our social and business goals with ML models’ behavior. We believe that model monitoring, explainability, and feedback tools – like Arize, Fiddler, and Humanloop – will play the biggest role in the AI governance stack as engineers work to increase visibility and control over AI systems.
The role of incumbents
Across both AI governance and MLOps, many of the most promising solutions are very young companies – businesses founded in the last 1-3 years, often with less than 100 employees and limited capital raised. One key question in this emerging category is whether agile incumbents – such as broader GRC platforms and platform/cloud vendors that support the full ML lifecycle – will step in and provide adequate responsible AI tools.
While we expect to see GRC platforms start releasing AI governance products, we believe there will be opportunities for standalone AI governance platforms to become enduring companies, particularly as the regulatory landscape and MLOps ecosystem matures. As MLOps tools become more sophisticated, we expect tighter integrations to develop between responsible AI platforms and MLOps platforms that facilitate monitoring and feedback – and expect that purpose-built AI governance tools will be better positioned to develop those integrations than broader GRC tools.
Beyond regulation: Responsible and ethical AI
Overall, while the regulatory momentum is driving much of the conversation on AI governance, we are also starting to see more proactive interest from enterprises in building AI systems that are not just compliant with regulatory requirements, but that fulfill their own standards of responsible and ethical AI. We are excited by the much-needed attention that this category is starting to command and are eager to speak to any founders working in the space.
Note: Fiddler, Zest, OneTrust, and Diligent are Insight portfolio companies.