Six Best Practices for Your First 90 Days as a Data Leader
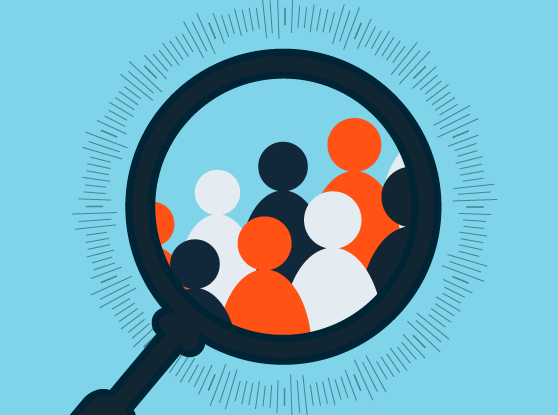
For newly hired data leaders, the first 90 days are crucial. For existing data leaders, it’s prudent to take a step back at least once a year, think about the business with fresh eyes and ask: What would a new data leader try to address in the next 90 days?
Regardless of title, CxOs of young and established organizations realize their ability to make excellent data-driven decisions is a must to achieve the company’s business goals. In the current resource-stretched climate, the CTO, CIO, or CxO often wears the data leader’s hat. This is especially the case at early-stage companies too small to have this position. But while the role of the data leader is still often shared, a data leader’s acumen and approach for data-driven decisions can be adopted by many leaders to have a significant impact on revenue and growth.
Key Insights
Every enterprise and business case is unique, but today’s data leaders have established a set of best practices to chart the digital pathway to profitability:
- Unify data and AI strategies
- Cultivate an open data culture
- Solve customer problems
- Capitalize on early opportunities
- Align stakeholders on priorities
- Inspire the team
Unify Data and AI Strategies
Young organizations often struggle to create meaningful data strategies. The key is to recognize where emerging trends and company needs intersect, and then create a roadmap to fulfill short- and long-term needs with technology that provides a competitive advantage. The data leader is aware of the importance of aligning data and AI strategies to better serve the organization’s overall mission.
A 2022 survey revealed that over half of the data executives surveyed expect AI to become “critical” to multiple facets of their business by 2025. While most companies expect to adopt AI technologies, many data leaders are looking even further ahead to more advanced forms of artificial intelligence. Techniques such as generative AI — capable of creating rather than simply predicting — has a fast-growing number of use cases.
Read more: 8 Tech Investors Share Predictions for 2023
A sophisticated AI model requires a modern data stack. Companies that can invest in their data infrastructure will be able to more successfully capitalize on emerging AI techniques. A full-stack approach to ETL (extracting, transforming and loading) data, creating data lakes and lakehouses and de-siloing data architectures can give companies a real competitive edge.
Similarly, powerful AI needs DevOps and MLOps tooling to support it. As the role of AI grows within an organization, data leaders will need a more robust foundational toolset if they want to identify which features of their data provide signal (a process known as feature engineering). This also holds true if they want to improve model training, deployment, and monitoring. Additionally, not all MLOps stacks are created equal — AI working with unstructured data requires different pipelines than those working with structured data. The same distinctions exist for generative versus predictive AI.
Today’s data leaders are seeing these AI trends play out in real-time and are adapting their enterprises to take advantage of what AI can offer for data-driven decisions and strategies. This is important not only to promote growth and accelerate innovation but to build resilience during periods of austerity. With the global economy in a period of stagflation (stagnation and inflation), the ability of business leaders to extract value from data could be a deciding factor in how well a company weathers the storm.
Cultivate an Open Data Culture
How a strategy is defined is up to leadership, but how well it is executed is often a function of company culture. Executives are reshaping their organizations to become more data-oriented, but the vast majority still point to culture as the greatest challenge in becoming a data-driven organization.
Successful data leaders hit the ground to build a data-driven culture by identifying stakeholder needs and responding to them with data-driven decisions. Bear in mind that the ultimate stakeholder is the customer. Organizations invest heavily in research to identify who their customers are, what their needs are, and how they differ by region and culture. Expanding these data sources and creating channels where data can be translated into actionable insights is a fundamental part of a data-driven culture.
The organization itself has hurdles to overcome: diversification of customer base, changing business priorities, and infrastructure and scalability concerns. External regulators demand certifications and scrutiny to ensure compliance with standards.
To succeed amid this tangled web of expectations, data leaders have established a practice of identifying objectives that are mission-critical to meet — those actions or failures that could cause a loss of stakeholder confidence.
Solve Customer Problems
Without data, there is no visibility in customer buying patterns, adoption, and behavior. For SaaS companies in particular, identifying a common enterprise data model is foundational to learning about their customers and fulfilling core business responsibilities. This is especially critical in the wake of multiple mergers and acquisitions, when customers may have to deal with multiple quotes, orders, contracts, and invoice formats that don’t match.
At the heart of all data is customer identity data, and your process for customer identity conflict resolution will be key. Unified data models allow businesses to integrate multiple data streams into enterprise resource planning pipelines. How to create and interpret information gleaned from data models might be beyond the technical capabilities of the data leader. However, data gleaned from the data model can provide an easily accessible wealth of information about how well the organization as a whole is able to fulfill customer needs.
Read more: 7 Habits of Effective Data Leaders
Merging these data streams provides much better visibility into customer buying behavior. This includes better insights on lead generation, sales conversions, annual recurring revenue (ARR), customer acquisition cost (CAC), net revenue retention (NRR), and more. More insights allow for better decision-making in every department of the organization. As with software quality and usability for an engineering department, this is a case of operational details determining strategic success. For companies growing through mergers and acquisitions, this is especially important.
Capitalize on Early Opportunities
Understanding where the business’s data organization performs against key performance indicators (KPIs) is an important part of a data leader’s job. Data leaders use a series of self-assessments to understand the strengths and weaknesses of their organization’s data strategy and capabilities. To really deliver value, however, executives often chart a series of phases for their data strategy — the so-called “Crawl, Walk, Run” progression.
As with any “baby steps first” approach, the essential “crawling” phase sees the development of the initial capability by building out the data stack and analysis pipeline. Once a process is in place, leaders can advance to the “walking phase” — fixing the parts of the process that are present but broken. The good news is the switch from walking to running is the easiest, as process fixes eventually become process optimizations.
It’s also important that this process is iterative. At some point, a process can no longer be optimized. In strong data-driven organizations, there is a point at which the team will look for more advanced technology to build, introducing more capabilities than previous optimizations could accomplish. This is also where the data leader’s leadership in building a data-centric culture, their attention to instituting policies for governance and security, and their investment in technologies such as generative AI and MLOps pay off.
This step is important for new data leaders. The average tenure of a data leader is 30 months, significantly shorter than for other executives. Data leader turnover, driven by a gap between expectations and results, often happens because leaders do not seize these early opportunities. This applies equally to data leaders hired to build data departments, and to those hired to make structural architectural changes (especially after a big data breach). Data leaders that fail to deliver demonstrable improvements — both in the department’s operations and overall business impact — struggle to make it past the two-and-a-half-year mark.
Align Stakeholders on Priorities
Balancing a host of competing priorities — investing in emerging tech, building culture, and process optimization — is difficult. Today’s data leaders have adapted by creating a prioritization framework along two axes:
- Importance to the business
- Difficulty of implementation
Understandably, data leaders often pursue the low-hanging fruit first, consisting of high-priority and low-difficulty items. Although those characteristics seem mutually exclusive, there are changes data leaders can make to secure easy wins. Achieving success for these items can be as simple as instituting transparency policies to keep the board updated on data-driven processes, such as the company’s data privacy and security efforts.
In 2021, less than half of surveyed corporate boards received reports about cybersecurity risks. Another survey revealed progress continued to be slow — only 37% of surveyed board members felt confident that their company was secure. Providing transparency and clear reporting can be on the lower end of the required-effort scale, while it boosts the board’s confidence in the company’s data initiatives and security posture.
Data leaders can use clear reporting to secure buy-in from board members and other stakeholders, demonstrating that their leadership and priorities are leading the company to a better place. Creating this continuous feedback loop helps keep board members, other executives, vice presidents, directors, and even customers, on the same page. It also smooths the way for future initiatives.
Inspire the Team
Finally, every data leader is a team leader. Inspiring the data team to reach new heights provides a force multiplier for growth. Storytelling using data and visuals can serve as a way to lead by example to inspire the team, ultimately changing the company’s trajectory from being a follower to becoming a leader — even a disruptor — in an industry segment.
Investing in technologies like generative AI not only unlocks new capabilities for the company but also provides opportunities for employee growth. It creates better data scientists and engineers.
It also ensures that, as the company grows from early-stage to ScaleUp, the data department remains competitive from a talent standpoint. Data leaders today are capitalizing on the investment in new trends to attract diverse and capable data professionals.
Taking charge of an existing data department — or taking on the role of building one — is a daunting challenge. But data leaders are tackling that challenge with careful prioritization, an eye on the technological horizon, and attention to the intersection of customer problems and business opportunities. They identify low-hanging fruit and communicate their early successes. Make that your plan for your first 90 days, and you’ll be off to what everyone can see is a great start.